Tips on Using Driver Based Revenue Forecasting Models
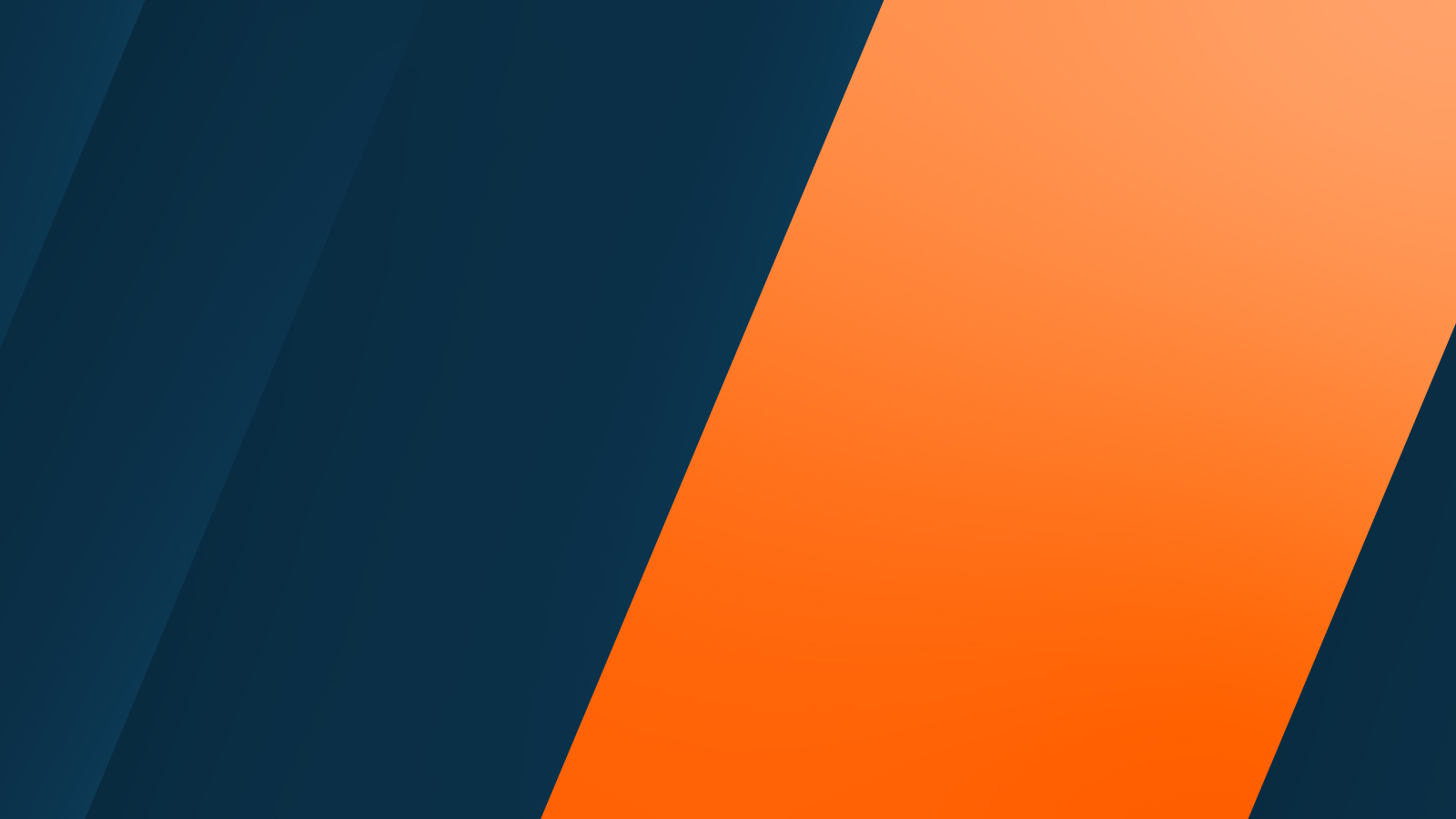
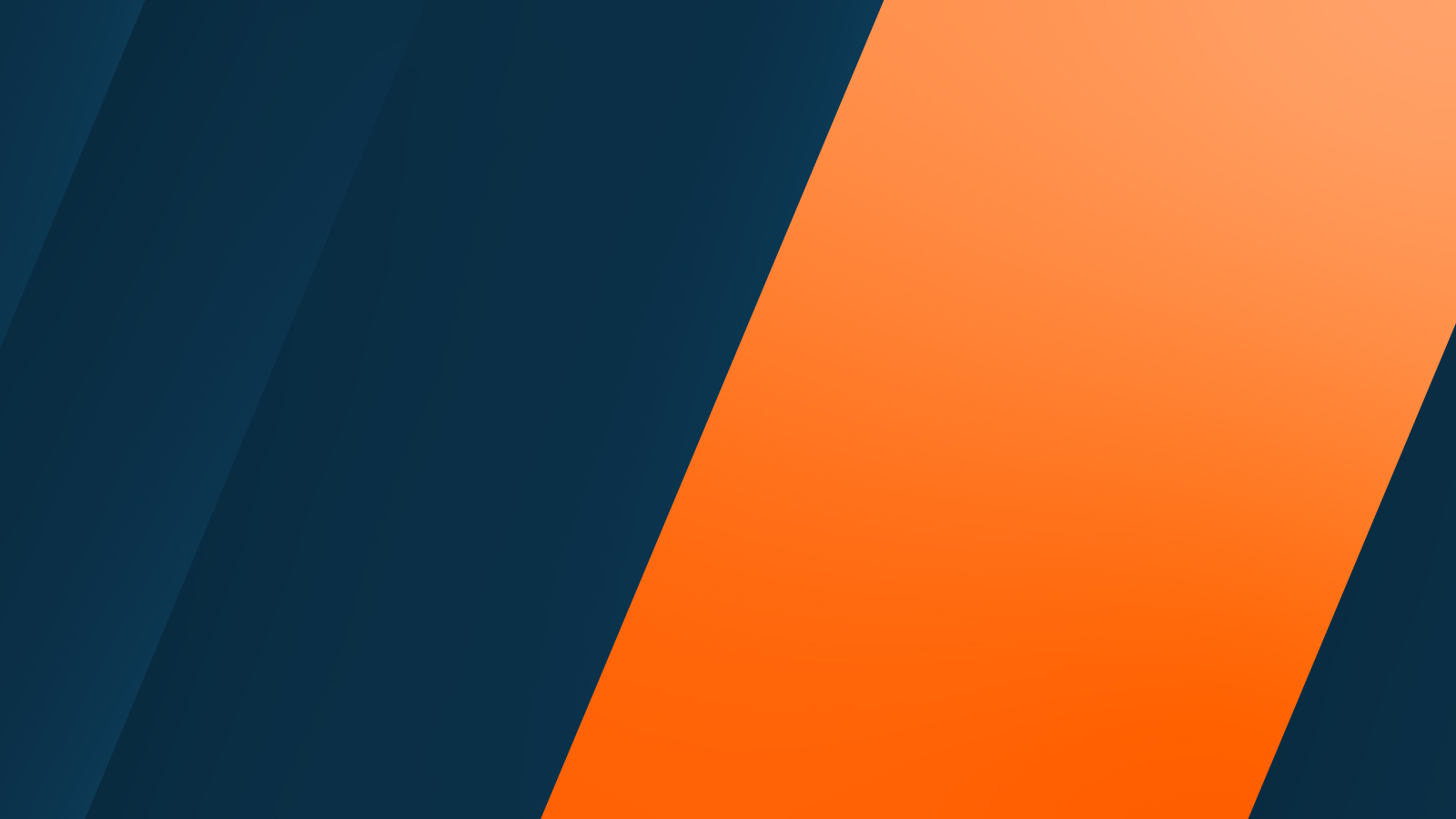
Somewhere tucked away in the back of a closet, we all have a box of family photographs. They were once in pristine condition, so the people and places portrayed in them were easily recognizable. But now they are blurred and faded so everything is indistinct. I tend to think of financial budgets in the same way. They are a great snapshot when they are first produced, but after just a few months, they no longer represent reality. Use them for setting incentive targets and send them off to your investors, but do not expect them to be that much use in managing the business during the coming year.
On the other hand, forecasting is dynamic rather than static as financial predictions are based on internal and external drivers using business rules to link line items, business functions, and time periods. So, if budgets are like snapshots, then forecasts are more akin to security cameras in that they are constantly sensitive to any movement in the internal and external drivers that impact future financial performance—and always ready to trigger alarm bells. Allow me to take this analogy a step further and suggest that perhaps the most critical aspect in building a dynamic forecasting model is identifying the drivers to incorporate—aka, knowing where to position those cameras.
The important thing is to select drivers that have a major impact on financial performance, and focus on those that are measurable and can be acted upon. This needs to be a collaborative effort so that everyone understands which pieces of the forecasting model they own and are responsible for maintaining. In my experience, if you try doing this in isolation, you risk ending up with a “black-box” that people will not buy into, and you will not realize the full value of adopting such an approach. Here is a five-point checklist for using drivers in forecasting models:
1. Maximize the use of drivers to model how revenue is generated
Revenue forecasting varies by industry, and could involve modeling various drivers such as average selling price, market size, market share, and market growth across multiple channels, organizational units, and geographies. Most businesses measure revenue at least monthly, but many measure it daily or even hourly. However, it is not always so straightforward, and may require an additional level of modeling where the number of sales calls or the amount of web traffic are important factors in revenue generation. Once reliable conversion rates are established and the average sales value is known, activity and enquiry levels can be used to forecast turnover, remembering that it may take many months before an enquiry turns into revenue.
2. Limit the use of drivers to variable operating expenses
Drivers such as input prices, productivity ratios, and even staff turnover have an important role in modeling the key variable expenses such as travel, staffing costs, and other demand driven consumables. They are not so applicable for forecasting line items that are essentially fixed. And it is best to avoid artificially pushing the logic if there is no obvious driver to bring into play. Use a traditional approach to forecasting these line items.
3. Focus on the practical rather than the theoretical
Modeling revenues and operating expenses are the two areas where drivers are most applicable to forecasting. The causal relationships between the amount of activity, such as the number of sales enquiries, and its subsequent impact on revenue, are well understood and can be reliably measured. The same cannot be said of customer satisfaction or consumer sentiment. Many companies periodically measure customer satisfaction, but unless the results can be used to model how changes in levels of satisfaction directly impact customer retention and subsequently revenue, my view is that incorporating it as a driver in a forecasting model is pushing the envelope a tad too far. Incorporating sentiment analysis is even more problematic as there is very little data available about the demographics of those active in social media, and no way of knowing whether they are even part of your target audience.
4. Avoid unnecessary complexity
Given the differences between industries and business models, it is not surprising that there are no hard and fast rules on the number of drivers to use in forecasting models. Restricting the use of drivers to only those elements of revenue or expense that have a major impact on profitability will obviously make it easier to maintain models. But anyone concerned that they may be overwhelmed by the complexity should remember that often a single driver, such as the number of sales orders, impacts the workload and resourcing needed across a number of different departments, such as order processing, dispatch, and receivables.
5. Include the critical external drivers
Whenever you visit a site protected by security cameras, you can be sure that some of them will be monitoring what is happening well beyond the perimeter fence. Good forecasting models should do the same by incorporating quantified figures for market size and market growth, and perhaps even some of the higher order factors that directly impact them. For instance, a company marketing infant nutrition might track the number of babies born each month in order to quantify the size of the available market in the immediate future.
I’ve already mentioned that the lack of transparency into driver-based forecasting models has been an issue that has slowed their adoption. It is still a problem when forecasting models are built in spreadsheets, as the logic is hidden deep in individual cells. However, platforms such as Anaplan have taken a different approach by keeping rules in a central store so users working with the model and managers wanting to review a model can quickly find and understand all the underlying logic. In the most recent release, navigating around a model has been made even easier with the introduction of model maps.
These provide visual representations of the connections between different elements of the plan—for example, how a driver, such as workforce benefit percentages, connects to workforce expenses. I guess you could say they do the same job as a wiring diagram that shows how security cameras are networked together.