Four tactics for smarter demand planning
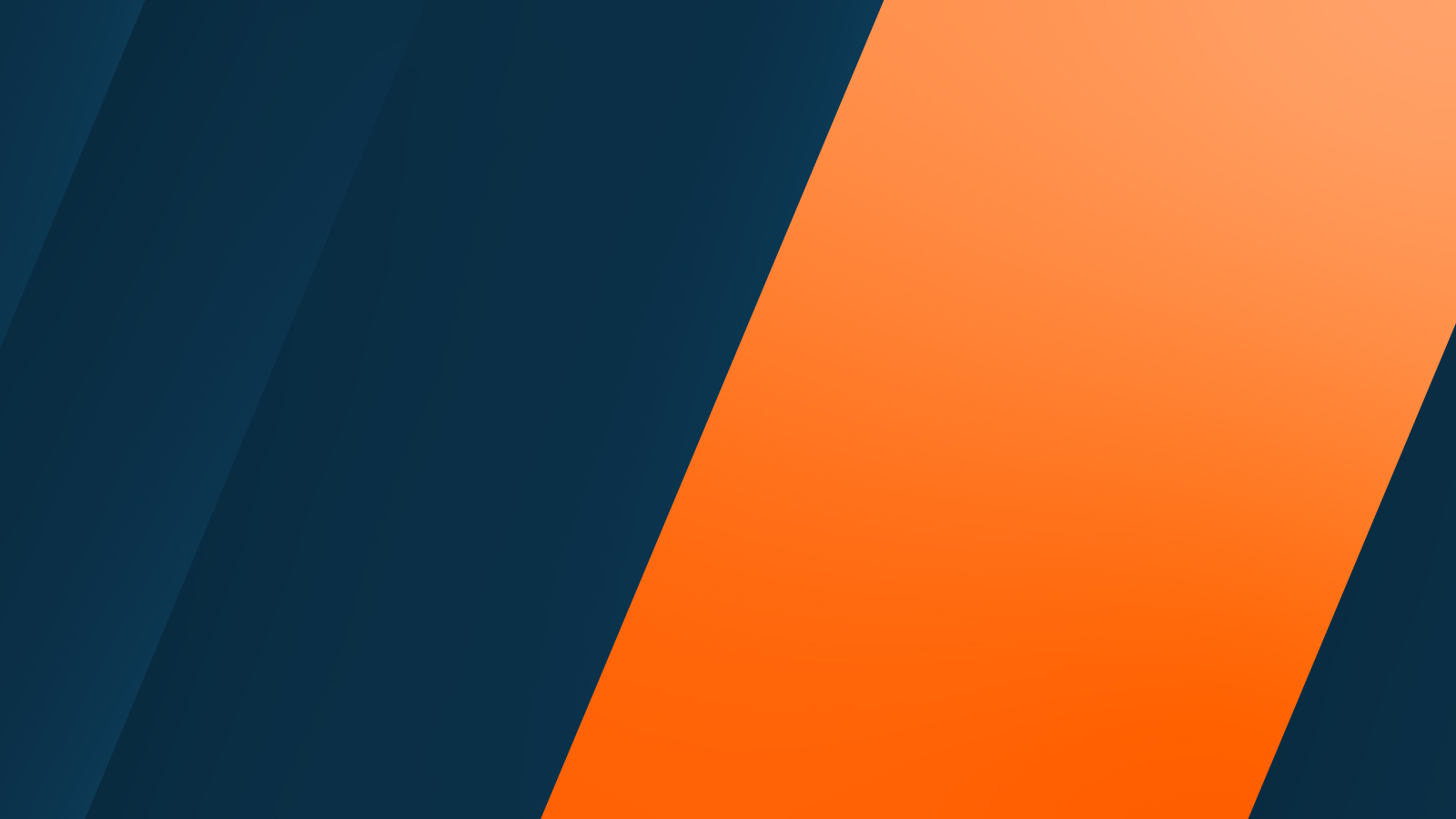
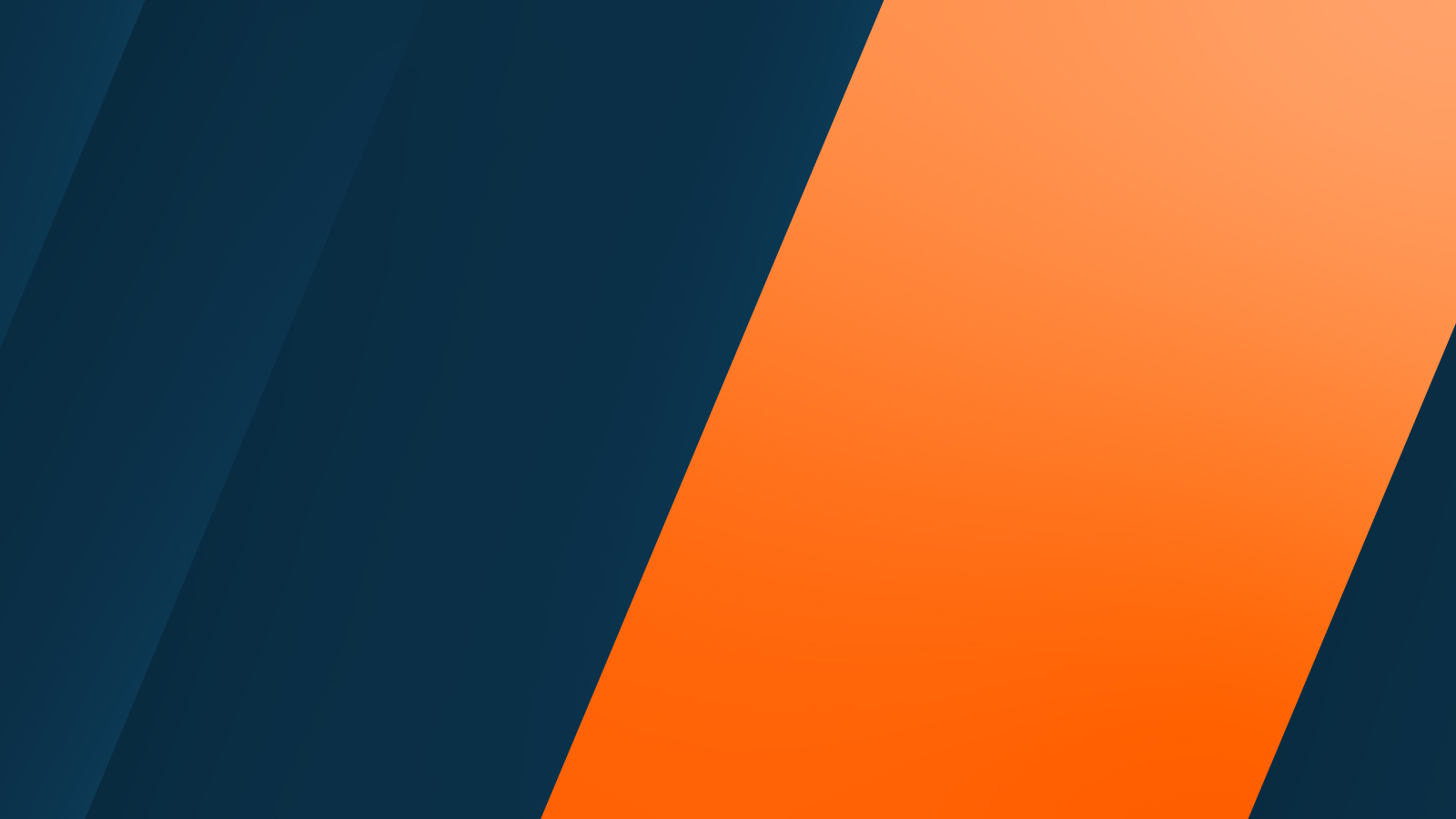
The pandemic spotlighted growing challenges to demand planning practices. We have guidance on how to do better in the new reality.
We can’t underestimate the impact of the COVID pandemic on demand forecasting, upon which so much else depends. During 2020, organizations found themselves in a rapidly changing environment that was more complex, volatile, and uncertain than ever before.
The past year’s events exposed many of the challenges that have been growing over the past decade—SKU proliferation, new distribution channels such as e-commerce, more compressed product lifecycles, and more demanding customers. As a result, the statistical forecasting techniques based on historical data that we had previously relied on no longer held up. Leaders found themselves looking for new sources of data and insights.
It boils down to this: Business leaders struggle to predict true demand and take appropriate action to address gaps. We focus too much on the forecast and not enough on the decisions we need to make.
Here’s the good news: Most companies now have an undeniable call to action to transform their supply chains and move past traditional demand planning and forecasting approaches. Here are four insights that can help you through the transformation.
1. Using artificial intelligence and machine learning to reshape forecasting
Forecasting doesn’t just assist in demand planning; it can also help the business shape demand and respond swiftly to changing market forces. To be most impactful, a forecast must not only be accurate, it must be generated early enough to allow the business to act effectively on its predictions. (For example, if a manufacturer needs four weeks to change production output, forecasting changes in demand only a week ahead doesn’t allow enough time to react.)
Artificial intelligence (AI) and machine learning (ML) have emerged as valuable techniques to address the need for speed in demand planning. But there are some challenges to consider:
- Extracting business-ready insights from ML platforms requires considerable cost and resources to develop, test, deploy, and maintain.
- Business users find it challenging to integrate AI/ML capabilities into their forecasting processes without heavy involvement from technical experts.
- Companies struggle to leverage the many sources of internal and external data to improve their forecasts.
- With siloed departments and the need for collaborative decision-making, many are unsure how to share AI-driven insights to drive informed decisions and turn decisions into actionable plans.
A senior planner from a beverage manufacturer told Anaplan recently: “We struggled with explainability, which is making the insights comprehensible to our business partners.”
Done right, the power of AI/ML is to build a better forecast and put that power directly in the hands of the business. That’s why Anaplan has developed PlanIQ, an intelligence framework that delivers advanced AI and ML capabilities for predictive forecasting.
PlanIQ makes ML-based forecasting accessible to demand planners so they can accurately predict demand, model different scenarios, uncover new trends, and speed finely tuned decisions. Planners can easily incorporate large data sets that span historical, internal, and external data. The ability to increase data volume and variety allows PlanIQ to deliver more accurate and continuously improving forecasts.
2. Rethinking forecast accuracy as a performance measure
Very few organizations would say that their forecasts were accurate enough when the pandemic hit; too much changed too quickly. In addition, many that we’ve talked to found themselves faced with conservative, risk-averse business cultures, which in turn introduced bias into the assumptions underlying the forecast and how it was interpreted.
Dealing with complexity at speed produced its own pressures. In some cases, planners found themselves faced with too much data to model effectively. Forecast inaccuracy—especially medium-to-long-term forecasts—became a fact of life, as did the need to get insights into what customers now valued and wanted to buy.
To overcome this, the greater emphasis must be placed on a collaborative planning process, with all parts of the business contributing and taking ownership of the aligned business outcomes.
Ultimately, the forecast is an input to the decision-making process; forecast accuracy simply measures what goes in. What comes out of the process is the most important.
Focusing on critical outcomes (such as On-Time-In-Full order fulfillment, inventory stocking levels, etc.) in the present should be the way to measure the forecast’s value, not just accuracy. We also see many customers using Forecast Value Add and Forecastability metrics to help focus their attention where it drives the most value.
As a senior strategic planner within a global CPG company told us: “Our forecasts had to work with other parts of the business and their metrics, including within their forecasting horizon—which could be as short as two weeks.”
3. Improving planning performance with updated segmentation
Another tool for dealing with complexity is segmentation: using customer and product attributes to divide them into groups, clusters, and associations that share common characteristics and behaviors. For example, a food manufacturer might have some perishable and other shelf-stable goods, which need different treatment in shipping and stocking.
Segmentation started with two key goals: 1) Simplify the process for the planner by allowing large groups of customers or products to be managed together, and 2) Enable differentiated policies for different groups, such as higher service levels for “A” customers.
However, the proliferation of products offered, along with a desire for ever more granular customer segmentation, has, in many cases, made legacy segmentation policies obsolete.
In addition to traditional methods, data science can help achieve an appropriate level of segmentation (a problem many CPG producers face). For example, ML is excellent at finding the links and associations in large volumes of data to automatically divide them into segments more quickly and accurately than humans could.
4. Moving from merely forecasting demand to shaping it
When the forecasting and planning process is made more efficient and accurate, the available time between insight and action increases. You move from forecasting demand in a reactive mode (“What can supply operations do to address gaps in the plan?”) to a proactive mode (“What can the commercial side of the business do to stimulate and shape demand?”). It becomes possible to run multiple scenarios and ask “what-if”?
At this point, planners can start to explore the impact of changing assumptions or actions, seeking the optimal outcome for the business. They are also better placed to pivot to rapid changes in the external environment.
A collaborative process is required to develop the plan—but turning insight into action also requires achieving consensus. That consensus is reached when your stakeholders trust the plan and acknowledge the limitations of any forecasting process in the current climate.