Defining Advanced Analytics vs AI and ML | Anaplan
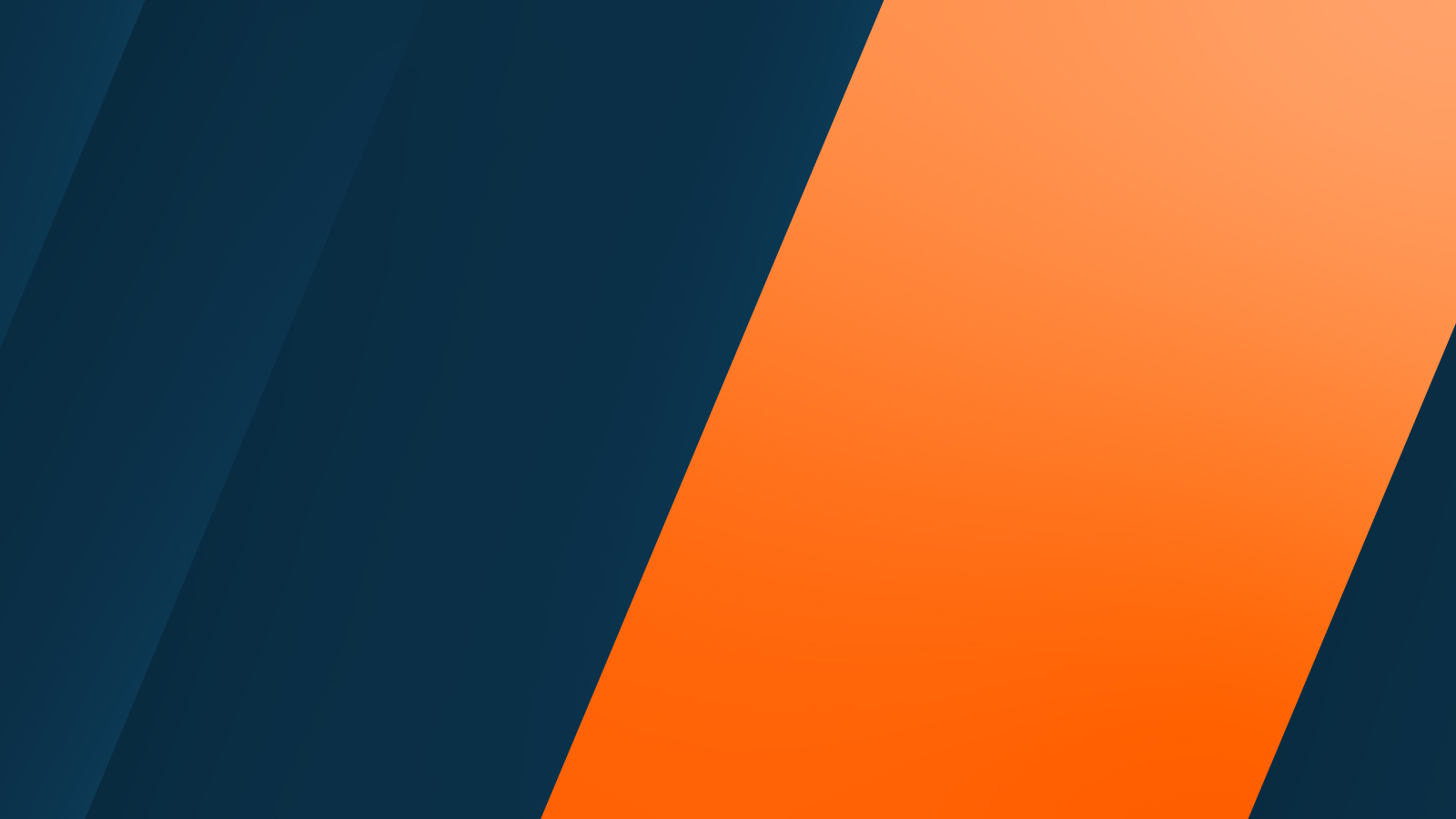
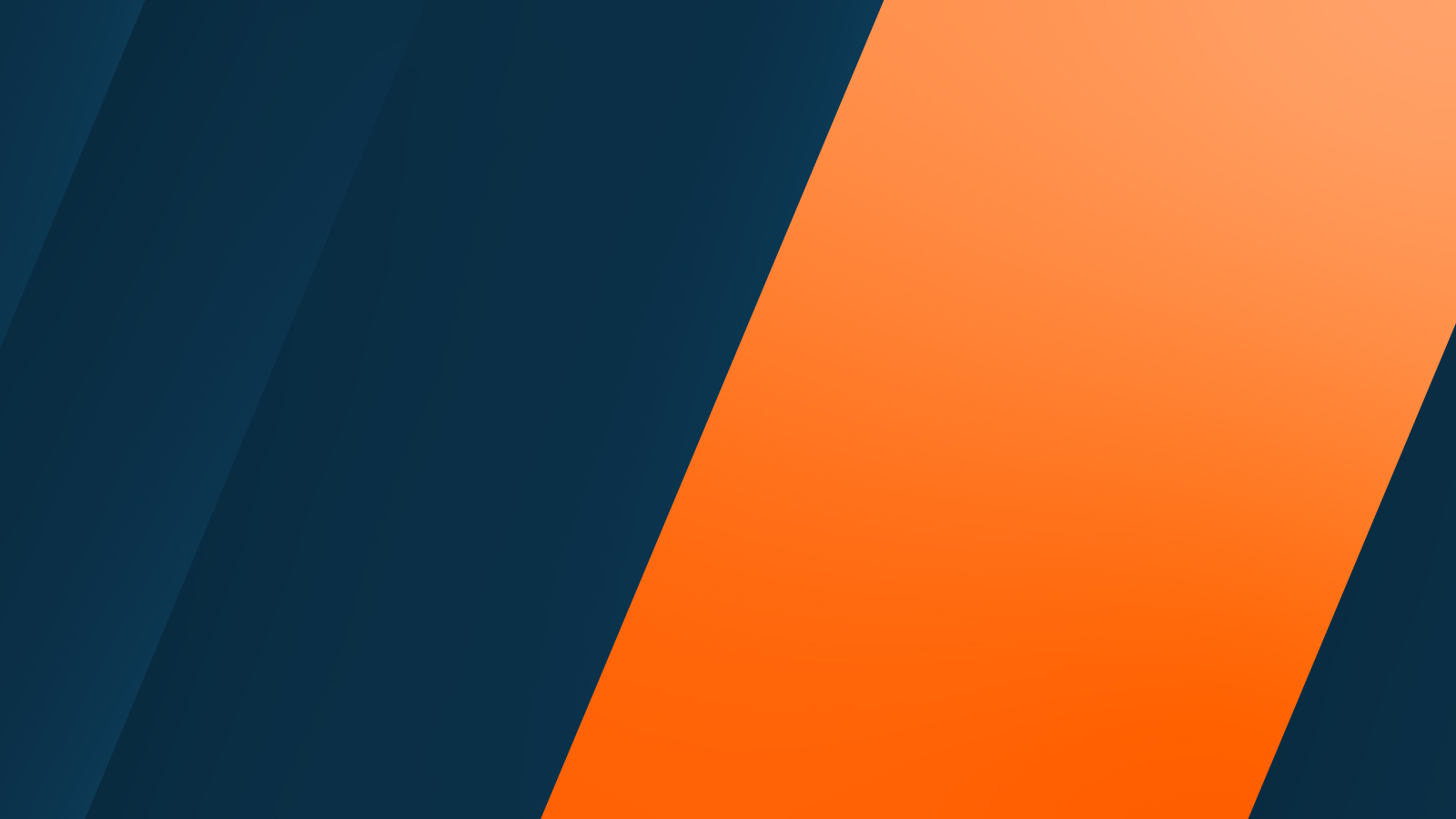
Technologies that not long ago were science fiction are now mainstream—and the possibilities look exciting. But jumping into that future isn’t easy. It’s full of buzzwords:
Artificial intelligence or AI. Machine learning. Predictive forecasting. Automated insights. Advanced analytics. Heard of them? Sure. But can you tell them apart?
Some of these terms have been used interchangeably, creating confusion that gets in the way of exploring which of the new technologies your company could benefit from the most. So let’s clear things up.
Definitions simplified
Artificial Intelligence or AI. Artificial means that it’s done by machines or devices, not a human. Humans have what’s called natural intelligence, meaning that organic beings collect and interact with data. Artificial means that a non-organic thing, such as a computer, collects and interacts with the data. Intelligence is defined as acquiring and applying knowledge, whether by an organic being or a computer.
That adds up to a simple explanation: Artificial intelligence is a machine or computer’s ability to acquire and apply knowledge.
Note that we said knowledge, not data. Here’s another distinction: Data is numbers, facts, lists, and statistics. Knowledge is data in context, and it’s gained through analysis and experience. Knowledge is data made usable. That’s what AI does; it makes data usable and applies it, sometimes in a new context.
AI is a broad term that covers many topics. First, let’s list some ways AI can be applied to everyday problems. (A single solution may fall into multiple categories.)
Natural language understanding. Personal voice assistants such as Alexa, Siri, and Cortana recognize speech, turning audio sounds into information they can acquire and use. These programs can learn to adapt to a regional accent or a mispronounced word in order to follow simple instructions, like playing music, turning on lights, or navigating to a location as you drive. (This technology allowed telephone trees to evolve beyond “press 6 to hear these options again, or press 0 to talk to an agent.”) Natural language understanding also works with text – for example, to translate languages or find relevant documents.
Digital vision. Tools like Facebook’s DeepFace and self-driving cars acquire information from digital images. For example, DeepFace recognizes which part of a photo is a human face and identifies it based on an AI tool that was trained using a database of known faces.
Other types of AI tools include chatbots, which can emulate human conversation, ask questions, and automate tasks in simple, structured ways (typically used in customer service), knowledge bots (the smarter cousins of chatbots, which can work in more open-ended ways to help you find desired information), and inference engines that apply logical rules to data, connect the dots, and deduce new information.
Now let’s define some major methodologies within AI.
Machine learning (ML). ML is when a computer or device acquires and interprets knowledge from a large amount of data and uses it in a way that improves its processes, with or without the aid of humans. ML uses the information it acquires to get faster, smarter, and more accurate over time, based on statistics and other mathematical applications. For example, if you provide a list of your existing customers, the ML model will be able to estimate the potential of others to also become your customers. Or you feed it stock market history, weather, political situations, and business data, and it comes up with intelligent math-based stock trade suggestions.
Predictive forecasting is a specific field in machine learning that deals with predicting the future values of a time series. Examples might be the demand for each of a company’s products, or the operational expenses of a company by business unit. Forecasting uses advanced methods to find leading indications of a future value, and to decipher dynamics in the historical data that hint what the future values will be.
Deep learning is a machine learning method based on artificial neural networks (computer algorithms arranged in patterns that mimic the way biological nervous systems communicate and grow). It’s one of the most cutting-edge and popular ML methods. It tries to identify patterns in data, both ones that can be easily revealed and hidden ones that only a complex algorithm will be able to detect. Deep learning techniques are used in many different use cases nowadays, including those listed above. They can be applied to problems based on labeled data (classification tasks, for example) as well as unlabeled data (e.g. anomaly detection or path optimization).
AI can super-charge analytics
The sophistication of the AI of a machine depends on how well it can logically apply information in new ways, with increasing effectivity, and even independently. Meanwhile, a different family of technologies – analytics or business intelligence (BI) – has also been getting more sophisticated and promising better answers for business questions. So where does advanced analytics fit into all of this?
Actually, all of these AI topics—and more—fit into advanced analytics. Traditional analytics or BI is a review of historical data that reports on past results. Advanced analytics uses AI and other techniques, including statistical and predictive forecasting, to look ahead. Put simply, traditional analytics asks, “What happened?” and advanced analytics asks, “What’s possible?”
How does all this affect planning?
AI and ML can make a big difference in business planning. Instead of basing (let’s say) this year’s sales forecast solely on last year’s figures, you could have a plan that takes projections for significant events into account—social or local events like festivals or sports championships, business events like mergers and other changes along the supply chain, regulatory changes, weather predictions and effects, seasonal demand, fads and trends, staffing issues—the possibilities are endless. (In fact, the many shocks of 2020 demonstrated how unreliable one year’s results can be in predicting the next year.)
The tool acquires the necessary information and applies it all by itself, leaving you free to review the results and come up with an intelligent response that keeps your company ahead of the competition.
More data, faster, can help with smarter predictions
Another factor in the rise of AI and ML is that today’s businesses have a lot more data available for planning. Advances in sensor, storage, and networking technologies make data cheaper and more abundant every year. Now an organization can install millions of sensors across all its operations, providing real-time changes in supplies, demand, workforce, global indicators, and much more.
For example, in 2020 the most important planning indicator for many businesses was the level of COVID-19 infections and counter-measures – data which no one foresaw as important just a few months before. AI-based forecasting can deal with vast amounts of data, and generate knowledge and insights for planning that are dynamic, agile and accurate.
Instead of plodding away trying to make a plan that foresees everything (or doesn’t), you could choose the most desirable result from an array of scenarios prepared for you, using criteria that you select. But it’s more than that—you won’t spend your time compiling and verifying data and trying to keep up with what’s happening. You and your team can get back to the work you really want to do. The status of the business is constantly updated and accurate, and that information is available on your nearest screen.
All clear?
When it comes to making the most of your data, there’s a lot to wrap your head around. Before you launch any new technology, whether you’re developing a time machine, a space program, or a better planning tool, you need to do some adequate preparation and study. You’ve just taken the first small step to a high return on your technology investment.