The fundamentals of building an accurate sales forecast
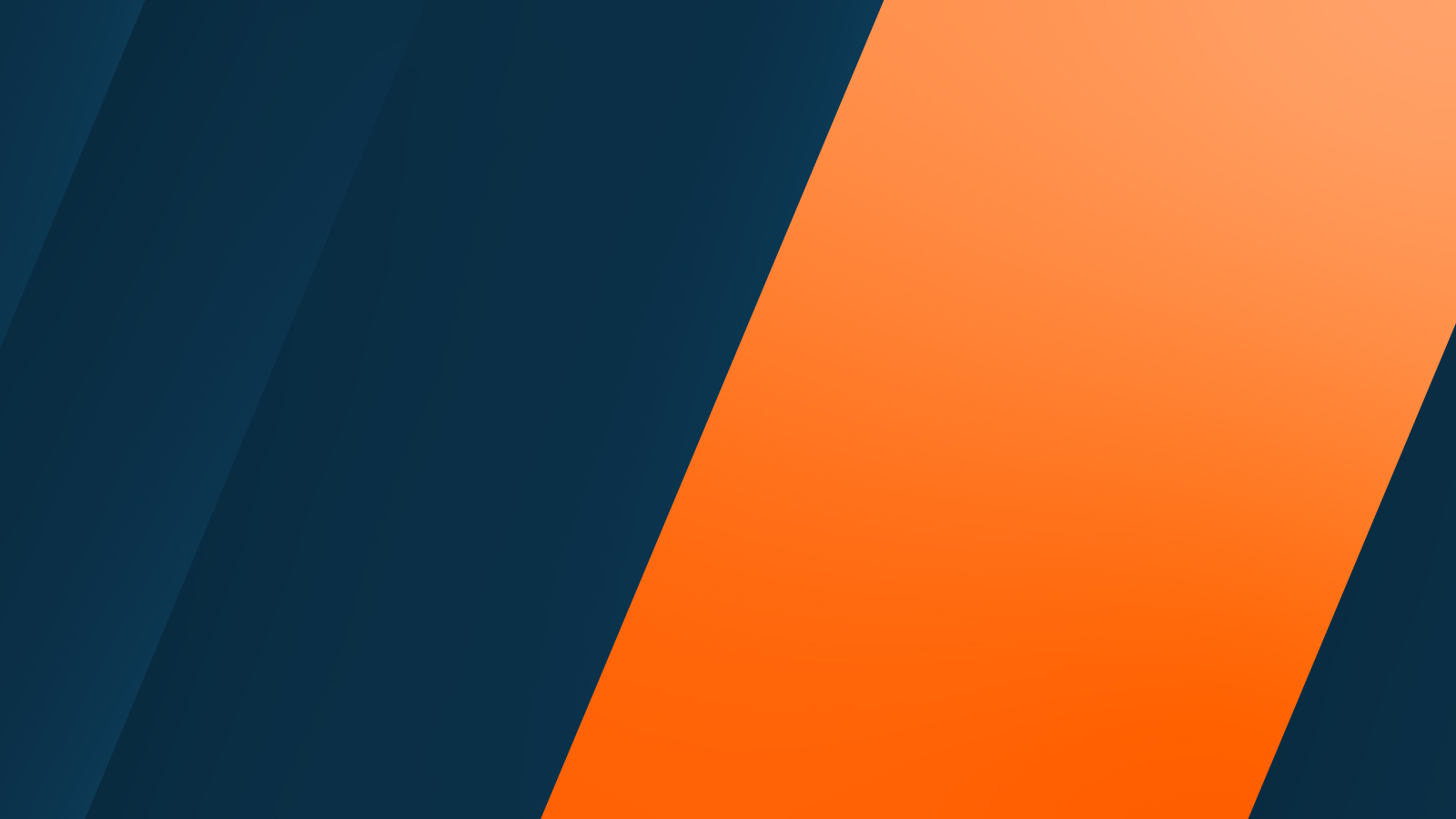
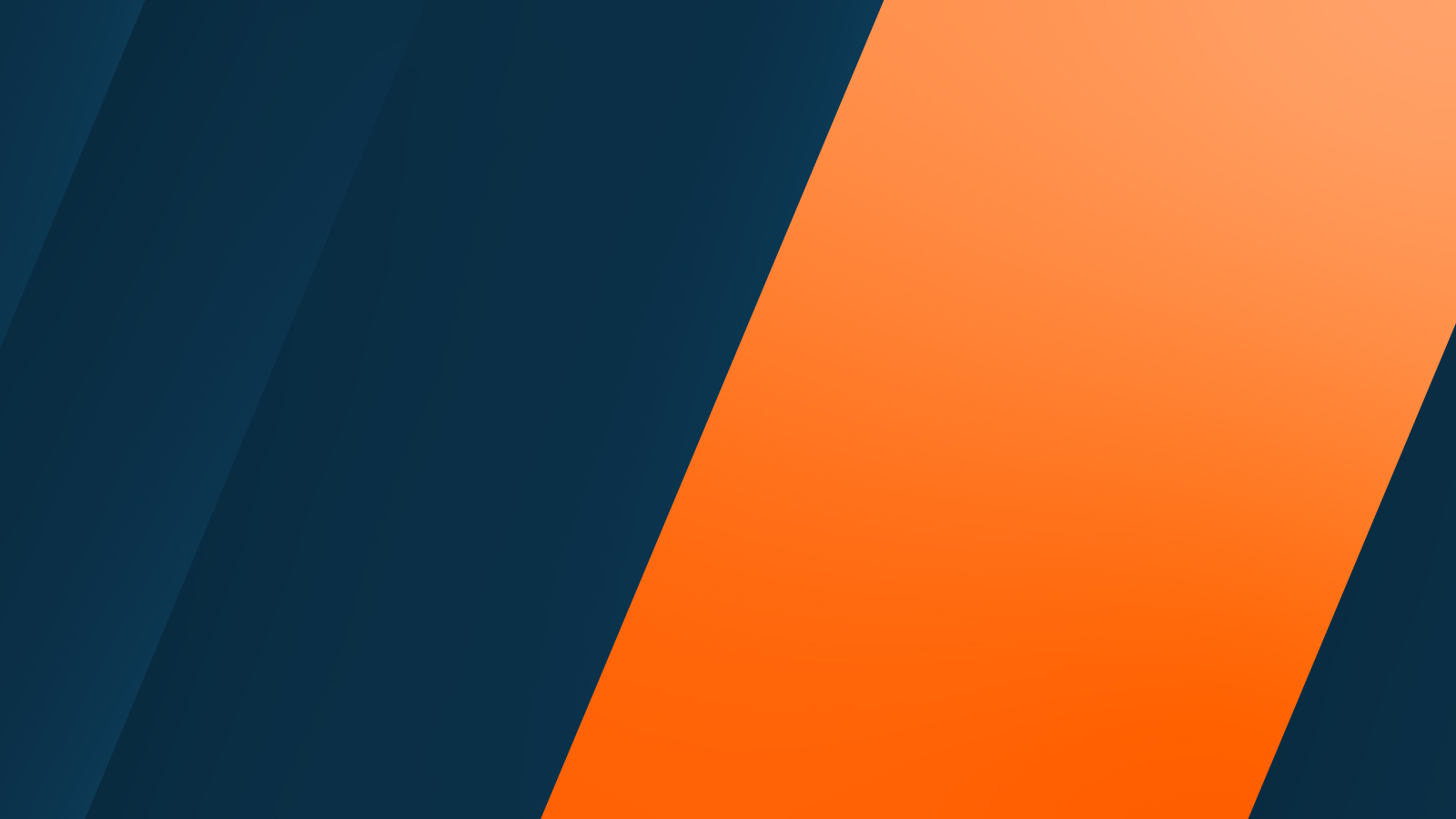
In a “Sales forecasting fundamentals” webinar hosted by the Sales Management Association, Abe Awasthi, Senior Manager at Deloitte walked through some recommended best practices for sales forecasting and discussed some roles that predictive analytics and machine learning are starting to play in the sales forecasting process. As Abe described, there is more disruption today than at any other time in modern history; intelligent companies are figuring out how to disrupt themselves before someone new comes in and does it to them.
The importance of sales forecasting
Sales forecasting is a measure of how the market will respond to your go-to-market efforts and a dynamic exercise that adds value across an organization. For example, finance relies on forecasts to develop budgets for sales capacity planning and hiring. Production uses sales forecasts to plan their cycles. Forecasts help sales ops with territory and quote planning, supply chain with material purchases and production capacity, and sales strategy with channel and partner strategies.
Unfortunately, at many companies, these processes stay disconnected, and it can produce adverse business outcomes. If information from a sales forecast isn’t shared, for example, product marketing may create demand plans misaligned with sales quotas or sales attainment levels. This leaves you with too much or too little inventory, or inaccurate sales targets — all mistakes that hurt the bottom line. Committing to regular, quality sales forecasting can help avoid them.
The components of a quality sales forecast
Because they touch so many other aspects of the organization, sales forecasts should be as dependable as possible. As Abe explained, the way to do this is to make sure sales forecasts exhibit three characteristics. Sales forecasts should be:
- Reliable. This depends on two things: data collection and analysis rigor.
- Collaborative. This includes not only workflow, which should include all necessary stakeholders, but also data quality, which comes from solid business processes and collective discipline — fundamentally collaborative activities.
- Actionable. This goal may seem obvious, but sales forecasts are often not presented in an actionable. To make them so, Abe offered two recommendations. First, provide “what if” modeling capabilities to sales reps, encouraging them to take a more holistic view of their deals and account plans. Second, deploy dashboards presenting different levels of insight to different stakeholders in the organization.
Ideally, some of these goals will reinforce one another — reliable pipeline data often comes from having a collaborative sales culture, for example. Similarly, the more reliable the data, the more actionable sales forecasts become.
The future of forecasting for sales: Predictive analytics is easier than assumed
Predictive analytics is already transforming many areas of business. Sales forecasting is no exception, yet terms like “predictive analytics” and “machine learning” can still be intimidating. To reduce this anxiety, Abe presented a short example explaining how predictive analytics improves forecasting:
A tech company asked Deloitte to produce a predictive model to improve sales forecast accuracy. To create this model, Deloitte leveraged the company’s available pipeline data from the previous few years, with customer and employee names removed. Deloitte then filled in the gaps in this data by extrapolating from historical trends using machine learning. Once it had done this, it then used the data to build two predictive forecasting models, one that calculated the probability that any given deal would close, and another that predicted the time frame in which that close would happen. When combined, these models provided highly actionable, very specific recommendations to the company’s sales team: “Push opportunity number five to qualified within the next 10 days or you’re going to lose it!”
Importantly, Deloitte was able to build these predictive forecasts in 8-12 weeks — a timeline feasible for many companies.
How to begin building a sales forecast
If a company is interested in transforming its sales forecasting, but not sure how to begin (data, collaboration, or predictive analytics?), it can help to assess some requirements, particularly the following:
- Stakeholders: Who is going to inform the forecast (sales reps, managers), and who will be using it (sales leadership, sales operations, finance, supply chain)?
- Time horizons: How far in advance will forecasting predict? The current quarter, rolling 12 months, or something else?
- Frequency of refresh: How often will the forecast need to be updated? This can depend on the stability of the market and the length of the sales cycle; the less stable the market or the shorter the cycle, the more often it will need to be refreshed.
- Granularity: How specific does the data need to be? Does it need to drill down to segments, cross segments, sub-segments, product lines, or even SKUs?
Grappling with these questions can help formulate a roadmap prioritizing the most essential elements for any situation.
Sales forecasting is a journey, not a destination
Sales forecasting isn’t a one-size-fits-all solution. The choices to make depend on the particulars of any one organization, including company culture, stakeholders, selling methodologies, and data needed.
From a larger perspective, it can be helpful to remember forecasting is as much a journey as it is a destination. The more forecasting is part of sales processes, the more organizations will pull in the right stakeholders, and priorities will become clearer.