ANAPLAN PARTNERS
Powerful partnerships that put our customers first
Driving the power of Connected Planning
Our Partners are critical to meeting the significant customer demand for Connected Planning. We’re proud to partner with many of the world’s leading experts to bring digital transformation to our customers.
PartnerAccelerate program
Partners with third-party verified expertise and experience across industry verticals and functional specializations support our customers in solving their business challenges.
Partner Portal
Visit Anaplan’s Partner Portal, where you will find all the tools and resources you need to succeed. Register deals, view opportunities, generate earned badges, and more, all on one single platform. Log in or request access today!
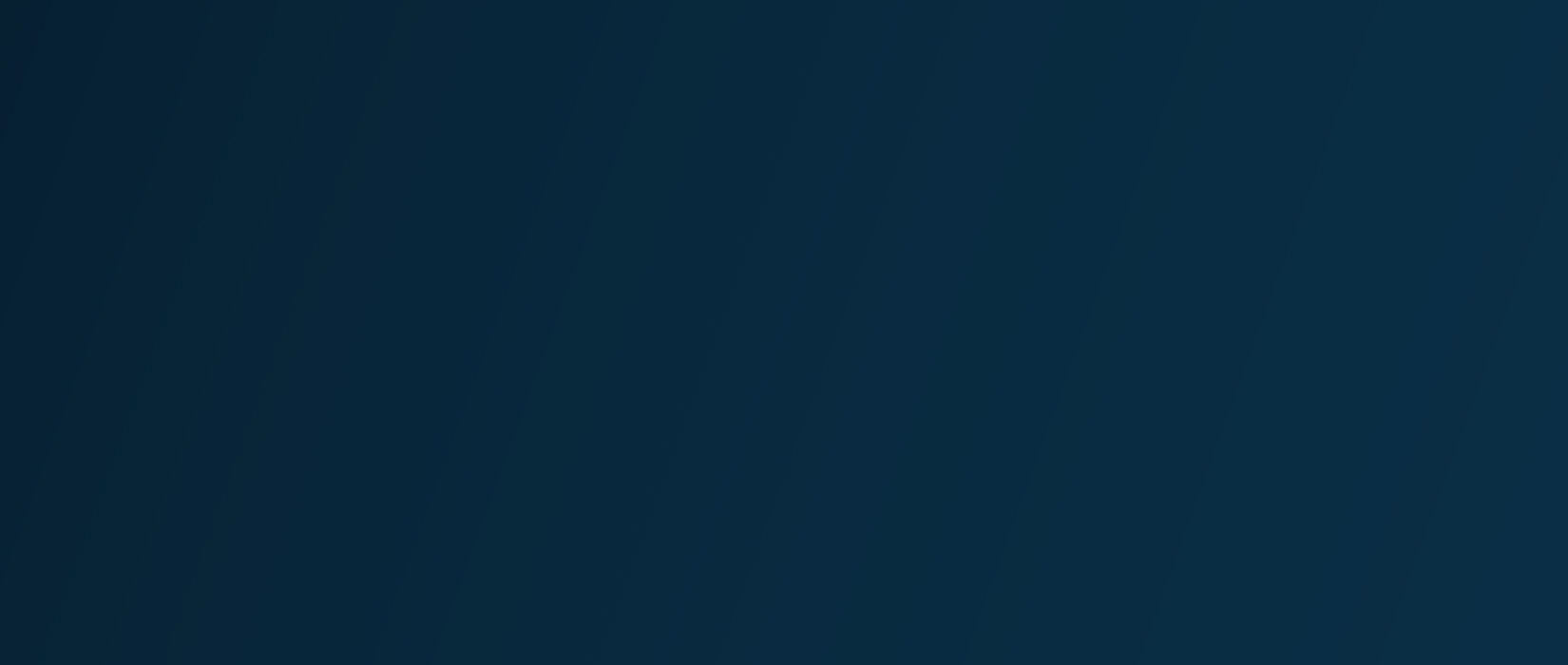
Partner badges in the PartnerAccelerate program
As part of PartnerAccelerate, Anaplan Partners can earn and display badges based on their areas of specialization. These badges enable Partners to differentiate their unique combination of expertise to potential customers and Anaplan sellers and earn benefits that include enablement, discounted and free training, and access to marketing co-funding.
Go-to-Market (GMT) Motion Badges
These badges recognize the primary ways Partners engage with Anaplan in the market. Partners earn GTM motion badges by accepting aligned Terms & Conditions, meeting defined requirements, and successfully completing the Delivery badge audit.
Specialization badges: based on expertise and Anaplan-specific experience in functional solution areas (Finance, Supply Chain, Workforce Planning, and Sales) and industries (Consumer, Manufacturing, Financial & Business Services, and Technology, Media & Telecom).
Note: More industry specialties will be added in the future. The PartnerAccelerate badge program also provides each Partner with the opportunity to create a customized set of Anaplan-specific credentials that support differentiating their market offers, empowering Partners to build and grow a profitable business with Anaplan.
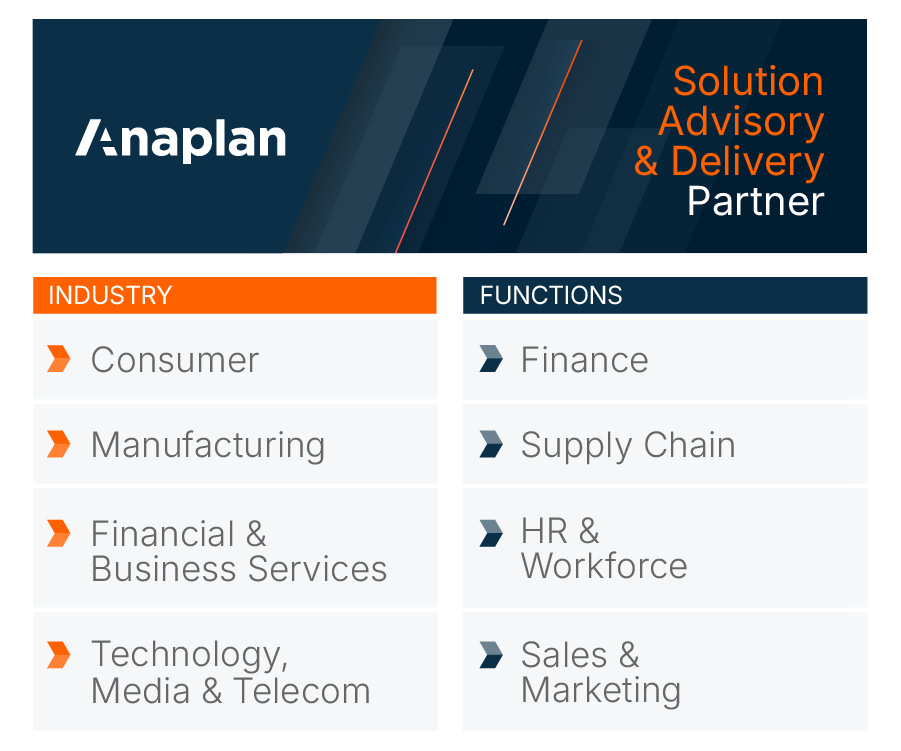
For information on how to qualify, please contact partner.help@anaplan.com Existing Partners can view more information in the PartnerAccelerate program guide. Link requires Partner Portal login.
Our Partners
Partners that participate in the PartnerAccelerate program are categorized and badged based on their areas of specialization.
Become an Anaplan Partner
The PartnerAccelerate program is designed to build growth and value for you and your customers through specialization badges. These badges are based on your expertise and experience in specific functional areas and industries. Specialization badges make it easy for customers to identify you as the Partner with the best skill set to meet their business needs.
Deliver greater customer value
Provide enhanced customer services, including pre- and post-sales customer success activities, to drive customer loyalty and retention and expand opportunities that raise your profitability.
Differentiate your organization
Inspire customer confidence, boost your credibility, and stand apart from the crowd by showcasing your proven expertise and experience with third-party, audited accreditations.
Receive rewards for performance
Accelerate your value and profitability with reward increases based on your performance across industries and functions, business expertise, and experience.